Use an Agile Approach to Define Business Needs & Develop an Effective ML Strategy
Many businesses and other organizations are considering how Artificial Intelligence (AI) and machine learning (ML) fit into their strategy. Algorithmia’s 2021 survey shows “an increase in prioritization, spending, and hiring for AI/ML. First off, 76% of organizations say they prioritize AI/ML over other IT initiatives.” However, simply assembling a data science team and building models is likely to lead to disappointment. Ultimately, the best model will only provide positive ROI if it produces actionable insights that you can use.
Define Your Business Goal
Understanding and defining which needs and goals could be addressed by data-driven insights is the most important investment. Before your organization builds anything, ask:
- What are you trying to achieve?
- What kind of decisions do you want to support?
- Which tasks are too complex to be addressed by traditional rules-based approaches?
- Is there some other challenge AI/ML could solve?
Even if there is an established goal, how to achieve it may be far from clear. A good example in the healthcare space is how fraud detection and prevention are becoming increasingly important. Reducing fraud-related losses is challenging because fraud takes many forms and fraud schemes are constantly evolving. Even if the goal is clear, the specific business problem we need to solve to achieve the goal may be far less well defined.
Narrow Focus: What are the specific requirements?
Once your organization has decided on a set of needs and goals, another set of questions are needed to better narrow the focus:
- What does success look like?
- Is it sufficient to classify historical data or does the business need predictions about future events or outcomes?
- What level of accuracy is required?
- How timely do insights need to be? In other words, how much time can pass between the moment relevant data is generated and the availability of actionable output?
Let’s take the optimization of delivery schedules as an example. A model that can generate the fastest and most fuel-efficient routes will still be a failure if it takes two days of data processing to deliver its output. In a delivery context, time is essential. With same-day deliveries no longer a competitive advantage, routes must be recalculated very quickly.
Similar examples from other domains illustrate that to build valuable AI/ML strategies, a clear understanding of the business context and relevant success criteria is essential.
Business Needs vs. Available Data
However, an understanding business of needs is only the beginning. Anytime we try to do something truly new and unknown, we are bound to encounter false starts and dead ends. This is especially true of AI/ML projects, which (except for some very well-defined standardized use cases with limited scope) contain significant unknowns and rarely follow a linear, predictable path. In addition to the usual technical and organizational uncertainties that all IT projects deal with, AI/ML efforts introduce uncertainty with the data, modeling algorithms, and the interactions of key variables.
As a result, what the business needs and what the data and technology make possible inform and constrain each other. Working out the best balance between them and discovering the best way forward requires rapid exploration and fast feedback to learn and adjust. In other words, an Agile approach that is tailored to the specific concerns of AI and ML efforts.
Refining Ideas with an AI Framework
An Ideation step is essential to making this process effective. It deliberately broadens the set of possible solutions by focusing on the overarching business goal—like reducing financial losses from fraud—and identifying alternative ways of meeting it. The objective of ideation is to create options.
The ideation process can be as simple or involved as the context warrants. Examples range from group brainstorming, to facilitated workshops with diverse stakeholders, to quantitative analysis of existing data.
Both the Ideation and Exploration phases should be short and focused. Setting a deliberate timebox is a good approach. The goal in these phases isn’t to build anything; it is to collect and assess ideas, so that the team can more rapidly converge on the most promising option(s).
Using a Lean Canvas is an effective way to document key determinants associated with various alternatives, such as:
-
- Uncertainties
- Any interesting patterns surfaced during exploration
- The state of the relevant data sources
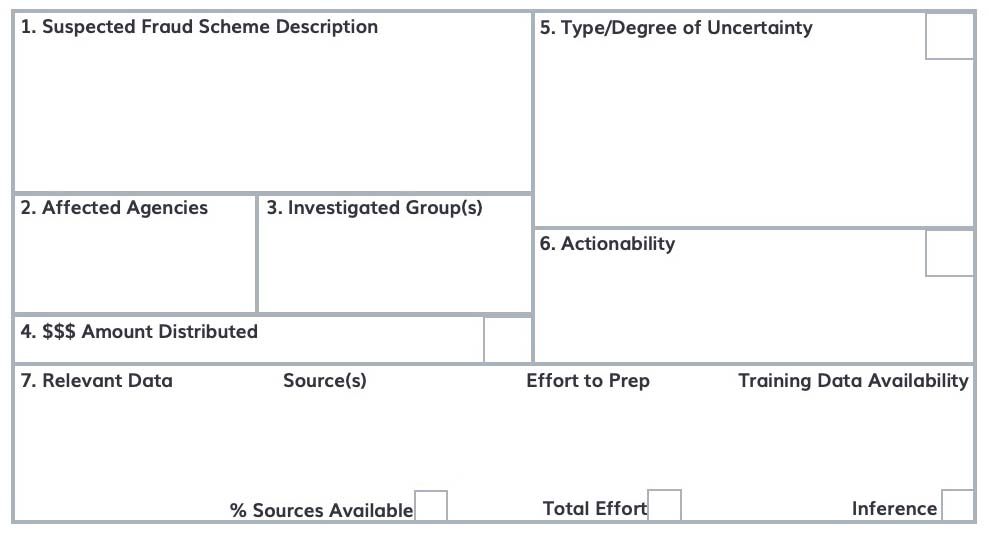
A lean canvas for an AI project to detect fraud waste and abuse.
Plans can also be developed for early user-facing prototypes or relevant user research based on the underlying business needs. As with any Agile approach, this work needs to be consistently validated through real feedback and adjusted as needed.
Avoid Jumping into AI Too Soon
One of the biggest risks associated with AI projects is jumping into development too quickly and committing to a solution approach without clearly understanding expected benefits or considering alternatives that may have higher ROI. By diligently exploring the set of possible solutions, teams can avoid getting stuck chasing a “perfect model” that may not be feasible or – worse – not deliver significant value to the business. These “perfect models” are an enormous risk. They waste time, budget, and effort, regardless of whether you’re pursuing market share or mission outcomes.
Maximize ROI with an AI Framework
It is essential to understand business needs, explore the options for solution approaches, and learn more about the possibilities before pursuing AI/ML strategies and solutions. This helps ensure that the ROI of the solution is maximized by focusing it on the right intersection of desired needs, technical feasibility, and the possibilities offered by the existing data. Each of these domains requires expertise, and the Agile approach that Excella has developed and is using for our clients today, harnesses that expertise for maximum benefit.